近日,实验室博士生丁泽源关于任务型对话的研究成果被Information Processing & Management (IPM) 期刊录用。IPM为中科院一区,CCF推荐B类期刊。
题目:A Plug-and-Play Adapter for Consistency Identification in Task-oriented Dialogue Systems
摘要:Task-oriented Dialogue system (ToD) has gained significant attention due to its aim to assist users in accomplishing various tasks. However, the neural network-based dialogue system is like a black box, which may lead to erroneous responses and result in an unfriendly user experience. To address this issue, consistency identification is proposed to prevent generating inconsistent responses. However, the existing consistency identification methods require frequent interaction with the knowledge base, making them susceptible to the introduction of noise during the knowledge base fusion process, ultimately leading to a decline in performance. In this paper, we propose a plug-and-play method for consistency identification, which can introduce external knowledge into the internal reasoning process of the pre-trained language model (PLM) without modifying PLM's structure. Additionally, we design a new fusion mechanism that effectively fuses the knowledge base information related to the current utterance, which helps the model avoid introducing noise from the irrelevant knowledge base. The experimental results demonstrate that our method achieves state-of-the-art performance on the consistency identification task, improving F1 scores by 2.9% absolute points over the previous methods. Finally, we investigate different knowledge base fusion methods and provide extensive experiments to show the advantages of our proposed method.
面向任务的对话系统(ToD)因其旨在帮助用户完成各种任务而受到广泛关注。 然而,基于神经网络的对话系统就像一个黑匣子,可能生成错误的回复从而导致不友好的用户体验。 为了解决这个问题,本文提出了一致性识别来防止生成不一致的回复。 然而,现有的一致性识别方法需要与知识库频繁交互,使得它们在知识库融合过程中容易引入噪声,最终导致性能下降。 在本文中,我们提出了一种即插即用的一致性识别方法,可以将外部知识引入到预训练语言模型(PLM)的内部推理过程中,而无需修改PLM的结构。 此外,我们设计了一种新的融合机制,可以有效地融合与当前话语相关的知识库信息,这有助于模型避免引入来自不相关知识库的噪声。 实验结果表明,我们的方法在一致性识别任务上实现了最先进的性能,与之前的方法相比,F1 分数提高了 2.9%。 最后,我们研究了不同的知识库融合方法,并提供了大量的实验来展示我们提出方法的优点。
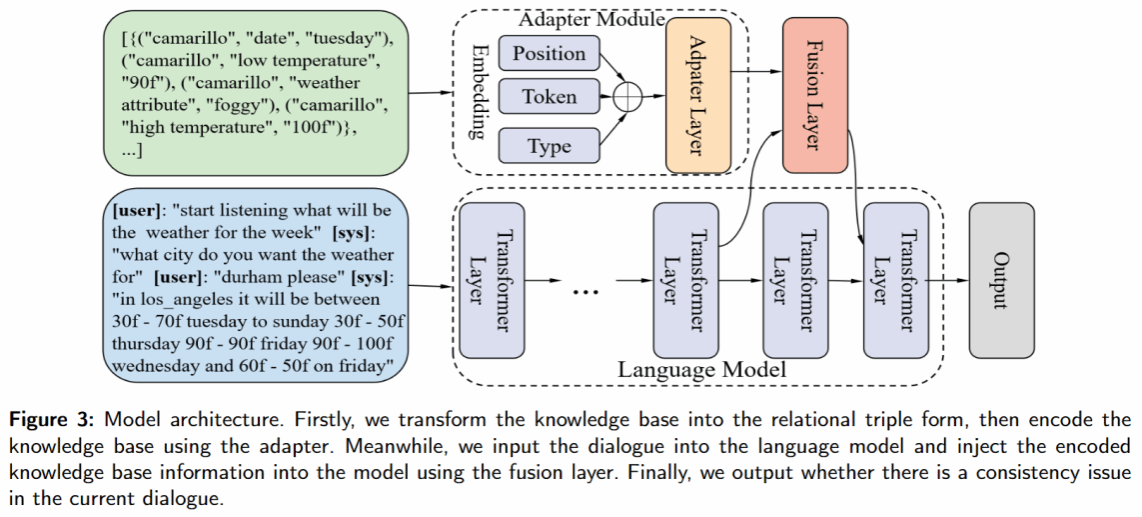