近日,实验室博士生张晓堃关于会话推荐的研究成果被国际顶级期刊 ACM Transactions on Information Systems(TOIS) 录用。TOIS是信息处理领域最权威的国际学术期刊之一,被中国计算机学会(CCF)推荐为数据库/数据挖掘/内容检索领域A类国际期刊,在信息检索,推荐系统和文本挖掘等领域享有盛誉。该期刊平均每年在全球仅录用50余篇学术论文。
题目: Bi-Preference Learning Heterogeneous Hypergraph Networks for Session-based Recommendation
摘要: Session-based recommendation intends to predict next purchased items based on anonymous behavior sequences. Numerous economic studies have revealed that item price is a key factor influencing user purchase decisions. Unfortunately, existing methods for session-based recommendation only aim at capturing user interest preference, while ignoring user price preference. Actually, there are primarily two challenges preventing us from accessing price preference. Firstly, the price preference is highly associated to various item features (i.e., category and brand), which asks us to mine price preference from heterogeneous information. Secondly, price preference and interest preference are interdependent and collectively determine user choice, necessitating that we jointly consider both price and interest preference for intent modeling. To handle above challenges, we propose a novel approach Bi-Preference Learning Heterogeneous Hypergraph Networks (BiPNet) for session-based recommendation. Specifically, the customized heterogeneous hypergraph networks with a triple-level convolution are devised to capture user price and interest preference from heterogeneous features of items. Besides, we develop a Bi-Preference Learning schema to explore mutual relations between price and interest preference and collectively learn these two preferences under the multi-task learning architecture. Extensive experiments on multiple public datasets confirm the superiority of BiPNet over competitive baselines. Additional research also supports the notion that the price is crucial for the task.
会话推荐(Session-based Recommendation)旨在根据匿名用户的行为序列来预测其下一次购买的商品。大量研究表明,商品价格是影响用户购买决策的关键因素。遗憾的是,现有的会话推荐方法仅旨在捕捉用户的兴趣偏好,而忽略了用户的价格偏好。事实上,主要有两个挑战阻止我们建模用户的价格偏好。首先,价格偏好与多种商品特征(即类别和品牌)高度相关,这要求我们从异质信息中挖掘价格偏好。其次,价格偏好和兴趣偏好是相互依存的,共同决定用户的选择,这就要求我们在意图建模中同时考虑价格偏好和兴趣偏好。为了应对上述挑战,我们提出了一种双偏好异构超图网络(BiPNet)。具体而言,我们设计了具有三级卷积的定制异构超图网络,以从物品的异构特征中捕获用户价格和兴趣偏好。此外,我们设计了一个双偏好学习模式来探索价格偏好和兴趣偏好之间的相互关系,并在多任务学习架构下同时学习这两种偏好。在多个公共数据集上进行的大量实验证实了BiPNet在会话推荐上的优越性能。进一步的研究也证实了建模用户价格偏好对会话推荐的重要性。
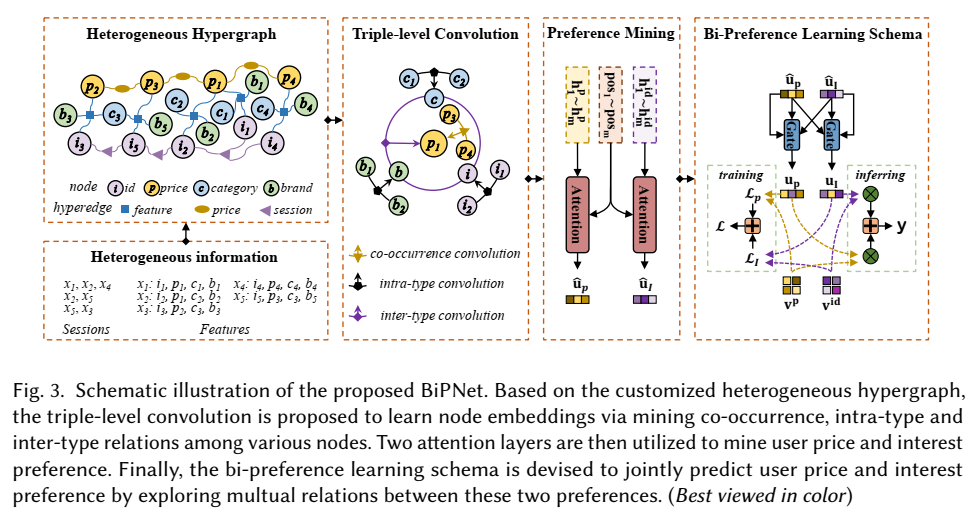