2020年10月14日实验室举行了博士留学生Michael的线上博士答辩会,Michael顺利通过博士答辩,获得了博士学位。Michael博士期间主要从事基于社交媒体的用户心理健康研究,分别从大五人格预测、抑郁倾向检测和自杀倾向预测等维度开展了社交媒体用户行为的分析与挖掘工作,取得了较好的科研成果,成果发表于国际SCI期刊,获得相关领域研究者的广泛关注。他的博士论文题目为《Early Prediction of Mental Health and Personality Based on User Social Behaviour in Social Media》(基于社交媒体用户行为的心理健康及人格预测研究)
论文摘要如下:
Social media has emerged as a novel communication paradigm in which the prediction approaches for mental health and personality are exploited to promote the improvement of social support services to social media users. Mental health is becoming an increasingly concerning topic in modern society. Depression is viewed as the largest contributor to global health disability, especially among the youth generation. In many countries, however, it is still under-diagnosed and left without any adequate treatment. Depression can lead to a serious self-perception, suicide ideation, and, at its worst, even to suicide completion.
The social stigma surrounding depression and suicide ideation prevents many affected individuals from seeking appropriate professional assistance. As a result, they turn to less informal resources, such as social media, where they seek social support. Early detection of mental disorders in social media can prevent at-risk-individuals from shifting to more severe mental disorder stages. According to existing studies, highly efficient methods for mental health analysis are based on conventional personality theories studying the association between personality and psychological factors that correlate with mental health. Thus, the importance of personality to mental health requires an accurate understanding of an individuals’ behaviour in some specific nonclinical environment.
The principal objective of our dissertation is to identify predictive markers of mental health and personality on two social media datasets using computational methods to improve the social support applications and early warning systems in social media. We focus on three research problems related to methodological improvements in the prediction of mental health and personality in social media. We begin with the review of the literature related to the prediction of mental health and personality and its reflection on social media.
After that, we examine users’ posts in Reddit social media forums to detect any factors that may reveal the depression attitudes of relevant online users. For such purpose, we employ the Natural Language Processing (NLP) techniques to extract the features and train the machine learning approaches and evaluate the efficiency of our designed method. Our validation processes report that depression has a significant impact on the language usage of affected individuals. Furthermore, we demonstrate that higher predictive performance is hidden in a proper feature selection and their multiple feature sets for mental health prediction in social media. The strength and effectiveness of the combined features (LIWC+LDA+bigram) are most successfully demonstrated with the Multilayer Perceptron (MLP) classifier resulting in the top performance for depression detection reaching 91% accuracy and 0.93 F1 scores. Next, we present hybrid deep learning architectures for the improvement of early detection of suicide ideation in social media. We explore the potential of LSTM and CNN deep learning models applied in multiple classification tasks and the implementation of their classifiers into a hybrid model to improve the language modelling and text classification performance. Our experimental results show the combined LSTM-CNN neural network model with 93.8% accuracy to outperform the state-of-the-art methods for early detection of suicide ideation in social media.
Our implementation of deep neural network architectures in social media can notably contribute to building the systems for early risk assessment and suicide risk prevention. Finally, we propose the Social Network Analysis (SNA) as a key technique for the improvement of personality prediction methods in social media. We clarify the relationship between users’ personality traits and their interaction behaviour in social networks defined by the social network properties. We explore the predictability of the Big Five model using social and psycholinguistic features. Our experiment shows that different personalities match different types of features. We conclude that using SNA features for personality prediction can result in a significantly higher performance with 78.6% accuracy.
Overall, our investigations reveal that methodological improvement in the prediction of mental health and personality in social media is an essential attribute for the improvement of future mental health detection systems to at-risk social media users. Besides, we show that the importance of personality to mental health requires an accurate understanding of an individuals’ behaviour reflecting their personality in the social media environment. Due to the privacy and ethical issues of social media users, there is still a lack of accessibility-related to connecting the mental health and personality into one large dataset. Bridging these two areas of research, we can develop a better understanding of real users’ intentions behind their posts to build more sophisticated and intelligent social support services and early warning systems in social media.
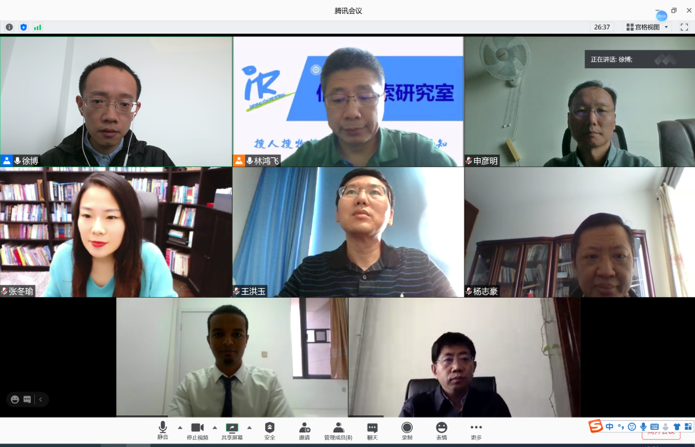