近日,NAACL 2025公布了录用论文列表,实验室共有两篇长文被录用。NAACL是计算语言学和自然语言处理领域的国际顶级会议,在学术界及业界都享有盛誉,在CCF推荐列表中认定为B类学术会议。
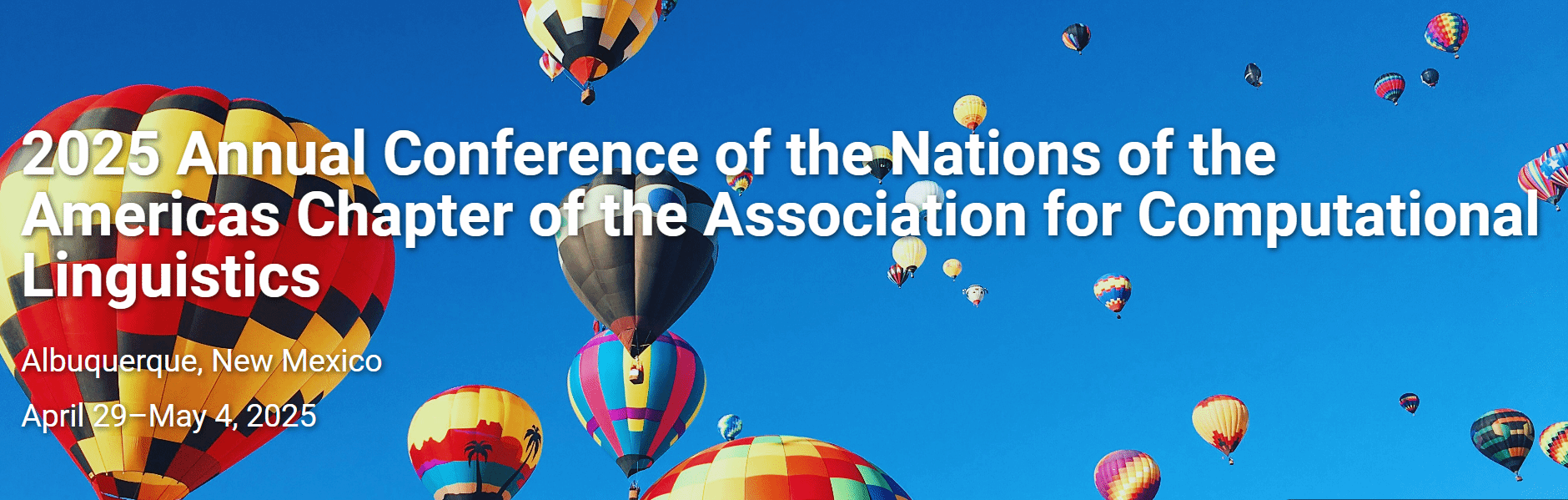
录用论文一:
题目:Commonality and Individuality! Integrating Humor Commonality with Speaker Individuality for Humor Recognition(共性与个性!将幽默共性与发布者个性相结合的幽默识别方法)
作者:博士生朱浩浩 等
录用类别:Main Conference
摘要:Humor recognition aims to identify whether a specific speaker’s text is humorous. Current methods for humor recognition mainly suffer from two limitations: (1) they solely focus on one aspect of humor commonalities, ignoring the multifaceted nature of humor; and (2) they typically overlook the critical role of speaker individuality, which is essential for a comprehensive understanding of humor expressions. To bridge these gaps, we introduce the Commonality and Individuality Incorporated Network for Humor Recognition (CIHR), a novel model designed to enhance humor recognition by integrating multifaceted humor commonalities with the distinctive individuality of speakers. The CIHR features a Humor Commonality Analysis module that explores various perspectives of multifaceted humor commonality within user texts, and a Speaker Individuality Extraction module that captures both static and dynamic aspects of a speaker’s profile to accurately model their distinctive individuality. Additionally, Static and Dynamic Fusion modules are introduced to effectively incorporate the humor commonality with speaker's individuality in the humor recognition process. Extensive experiments demonstrate the effectiveness of CIHR, underscoring the importance of concurrently addressing both multifaceted humor commonality and distinctive speaker individuality in humor recognition.
幽默识别旨在识别特定发布者的文本是否幽默。目前的幽默识别方法主要存在两个局限性:(1) 它们只关注单一的幽默特征,而忽略了幽默的多面性;(2)它们通常忽视了发布者个性的关键作用,这对于全面理解幽默表达至关重要。为了克服以上局限,我们提出了 Commonality and Individuality Incorporated Network for Humor Recognition(CIHR),一种新颖的幽默识别模型。CIHR旨在通过将多方面的幽默共性与发布者的独特个性相结合来增强幽默识别。CIHR 具有一个幽默共性分析模块,用于探索用户文本中的多方面幽默共性,以及一个发布者个性提取模块,用于捕获发布者个人资料中的静态和动态特征,以准确建模他们独特的个性。此外,CIHR还引入了静态和动态融合模块,以在幽默识别过程中有效地将幽默共性与发布者的个性相结合。广泛的实验证明了 CIHR 的有效性,强调了在幽默识别中同时解决多方面幽默共性和独特发布者个性的重要性。
录用论文二:
题目:Prototype Tuning: A Meta-Learning Approach for Few-Shot Document-Level Relation Extraction with Large Language Models(原型调优:一种基于元学习的大型语言模型少样本文档级关系抽取方法)
作者:博士生潘丁豪 等
录用类别:Findings
摘要:Few-Shot Document-Level Relation Extraction (FSDLRE) aims to develop models capable of generalizing to new categories with minimal support examples. Although Large Language Models (LLMs) demonstrate exceptional In-Context Learning (ICL) capabilities on many few-shot tasks, their performance on FSDLRE tasks remains suboptimal due to the significant gap between the task format and the intrinsic capabilities of language models, coupled with the complexity of ICL prompts for document-level text. To address these challenges, we introduce a novel meta-training approach for LLMs termed Prototype Tuning. We construct simulated episodes using data with relation types that do not overlap with the test corpus, fundamentally enhancing the ICL capabilities of LLMs in FSDLRE through meta-learning. To further enhance the effects of meta-learning, we innovatively integrate the concept of prototype into the fine-tuning process of LLMs. This involves aggregating entity pairs from support documents into prototypes within the prompts and altering the way of determining relation categories to identify the closest prototype. Experimental results demonstrate that our LLMs trained with this approach outperform all baselines. Our proposed approach markedly improves the ICL capabilities of LLMs in FSDLRE and mitigates the impact of relation semantic discrepancies between the training corpus and the test corpus on model performance.
少样本文档级关系抽取(FSDLRE)的目标是开发能够通过最少的支持示例推广到新类别的模型。尽管大型语言模型(LLMs)在许多少样本任务中表现出卓越的上下文学习(ICL)能力,但由于任务格式与语言模型内在能力之间存在显著差距,以及文档级文本的上下文提示复杂性,LLMs在FSDLRE任务上的表现仍然不尽如人意。为了解决这些挑战,我们提出了一种用于LLMs的新型元训练方法,称为原型调优(Prototype Tuning)。我们通过使用与测试语料库中关系类型不重叠的数据构建模拟训练情景,基于元学习从根本上增强了LLMs在FSDLRE任务中的上下文学习能力。为了进一步增强元学习的效果,我们创新性地将原型的概念整合到LLMs的微调过程中。这种方法通过在提示中将支持文档中的实体对聚合为原型,并将关系类别的确定方式转换为识别最接近的原型来实现。实验结果表明,采用该方法训练的LLMs在所有基线模型中表现最佳。我们提出的方法显著提升了LLMs在FSDLRE任务中的上下文学习能力,并缓解了训练语料库和测试语料库之间关系语义差异对模型性能的影响。