近日,机器学习领域的国际顶级会议NeurIPS 2024公布了录用论文列表,博士生卢俊宇关于有害模因检测的研究成果被Dataset & Benchmark Track录取为长文。NeurIPS被CCF推荐为A类国际学术会议,在学术界及业界均享有盛誉。其中,Dataset & Benchmark Track是发布高质量机器学习数据集和基准测试的论文平台,并为探讨如何优化数据集开发提供了交流论坛,被录用的论文将在NeurIPS主会上正式发表。NeurIPS2024的Main Track录用率为25.8%,D&B Track录用率为25.3%。
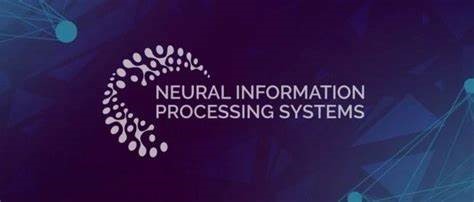
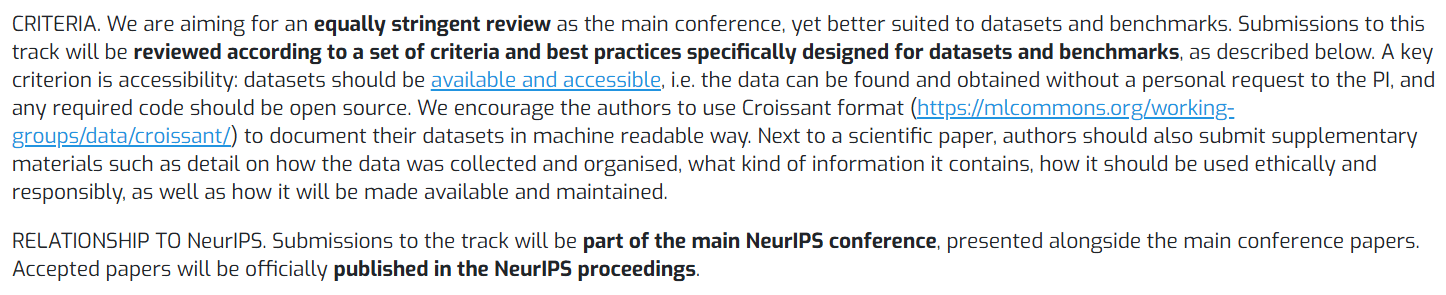
录用论文:Towards Comprehensive Detection of Chinese Harmful Memes
摘要:Harmful memes have proliferated on the Chinese Internet, while research on detecting Chinese harmful memes significantly lags behind due to the absence of reliable datasets and effective detectors. To this end, we focus on the comprehensive detection of Chinese harmful memes. We construct ToxiCN MM, the first Chinese harmful meme dataset, which consists of 12,000 samples with fine-grained annotations for meme types. Additionally, we propose a baseline detector, Multimodal Knowledge Enhancement (MKE), which incorporates contextual information of meme content generated by LLMs to enhance detection model's understanding of Chinese memes. In the evaluation phase, we conduct extensive quantitative experiments and qualitative analyses on multiple baselines. Experimental results indicate that detecting Chinese harmful memes is challenging for existing models, while demonstrating the effectiveness of MKE.
中文互联网上的有害模因日渐猖獗,然而由于缺乏可靠的数据集和有效的检测器,中文有害模因检测的研究明显滞后。为此,我们致力于对中文有害模因进行全方位检测。我们构建了ToxiCN MM,这是首个中文有害模因数据集,包含12,000个样本,并针对模因类型进行了细粒度标注。此外,我们提出了一种基线检测器——多模态知识增强(MKE),该方法融合了由大模型生成的模因背景信息,增强检测模型对于中文模因的理解。在评估阶段,我们对多个基线模型进行了广泛的定量实验和定性分析。实验结果表明,现有模型在检测中文有害模因方面面临挑战,同时证明了MKE的有效性。