近日,人工智能顶级会议(AAAI 2024)公布了录用论文列表,实验室博士生丁泽源任务型对话的研究成果被录取为长文。AAAI 是人工智能顶级会议,被CCF推荐为A类国际学术会议。
题目:From Retrieval to Generation: A Simple and Unified Generative Model for End-to-End Task-Oriented Dialogue
摘要:Retrieving appropriate records from the external knowledge base to generate informative responses is the core capability of end-to-end task-oriented dialogue systems (EToDs). Most of the existing methods additionally train the retrieval model or use the memory network to retrieve the knowledge base, which decouples the knowledge retrieval task from the response generation task, making it difficult to jointly optimize and failing to capture the internal relationship between the two tasks. In this paper, we propose a simple and unified generative model for task-oriented dialogue systems, which recasts the EToDs task as a single sequence generation task and uses maximum likelihood training to train the two tasks in a unified manner. To prevent the generation of non-existent records, we design the prefix trie to constrain the model generation, which ensures consistency between the generated records and the existing records in the knowledge base. Experimental results on three public benchmark datasets demonstrate that our method achieves robust performance on generating system responses and outperforms the baseline systems. To facilitate future research in this area, the code is available at https://github.com/dzy1011/Uni-ToD.
从外部知识库检索适当的记录以生成信息丰富的回复是端到端任务导向对话系统(EToD)的核心能力。现有的方法大多数额外训练检索模型或使用记忆网络检索知识库,这将知识检索任务与回复生成任务解耦,导致难以联合优化并且无法捕捉两个任务之间的内部关系。 在本文中,我们提出了一种简单且统一的面向任务的对话系统生成模型,它将EToDs任务重新构建为单个序列生成任务,并使用最大似然训练以统一的方式训练这两个任务。为了防止生成不存在的记录,我们设计了前缀trie来约束模型的生成,保证了生成的记录与知识库中已有记录的一致性。三个公共基准数据集的实验结果表明,我们的方法在生成系统回复方面实现了稳健的性能,并且优于baseline。
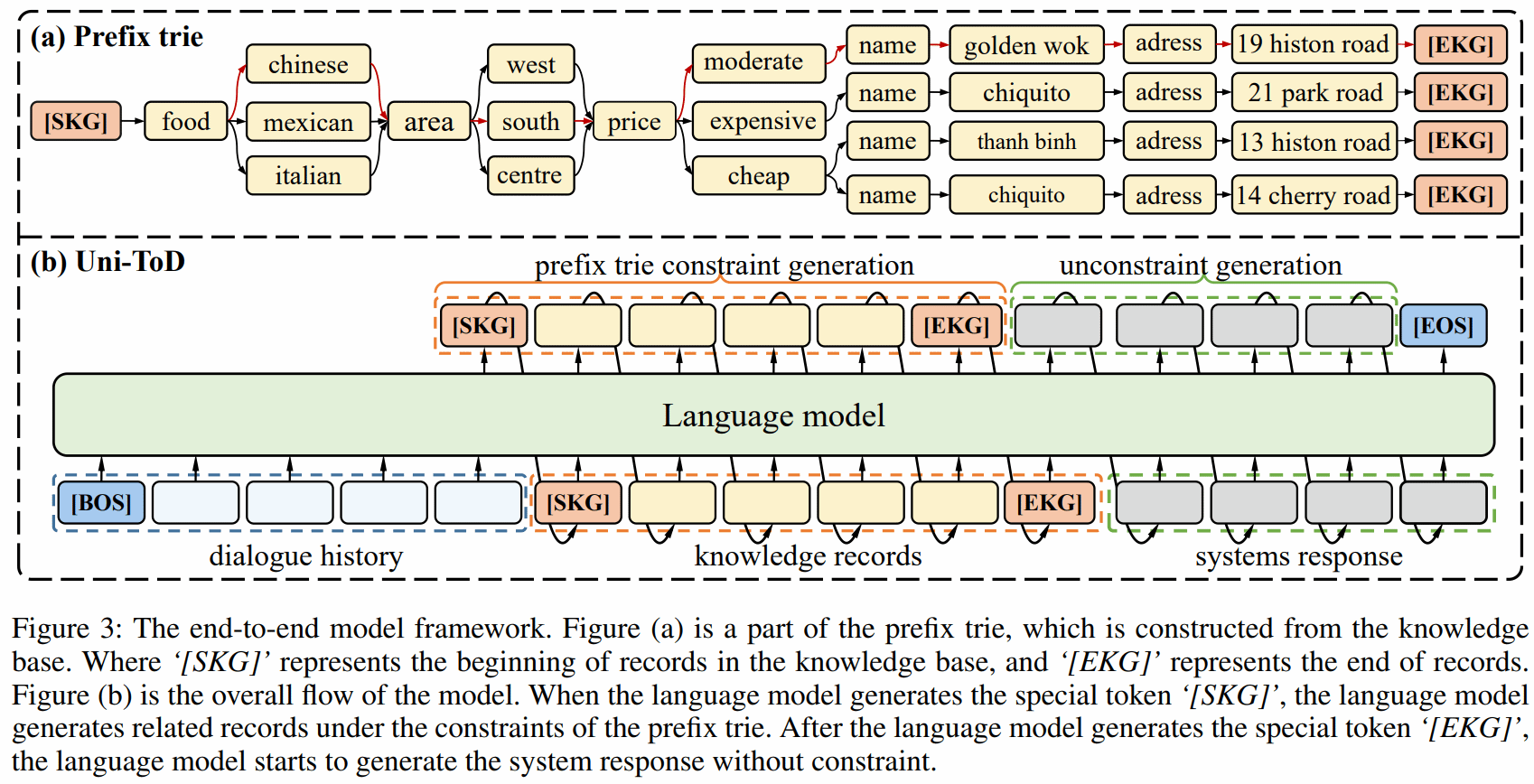